Model Risk Management (MRM) is a top priority for 2021. We have compiled the top six themes from discussions with senior industry leaders.
6 Key Takeaways from GFMI Model Risk Management 2021 Conference
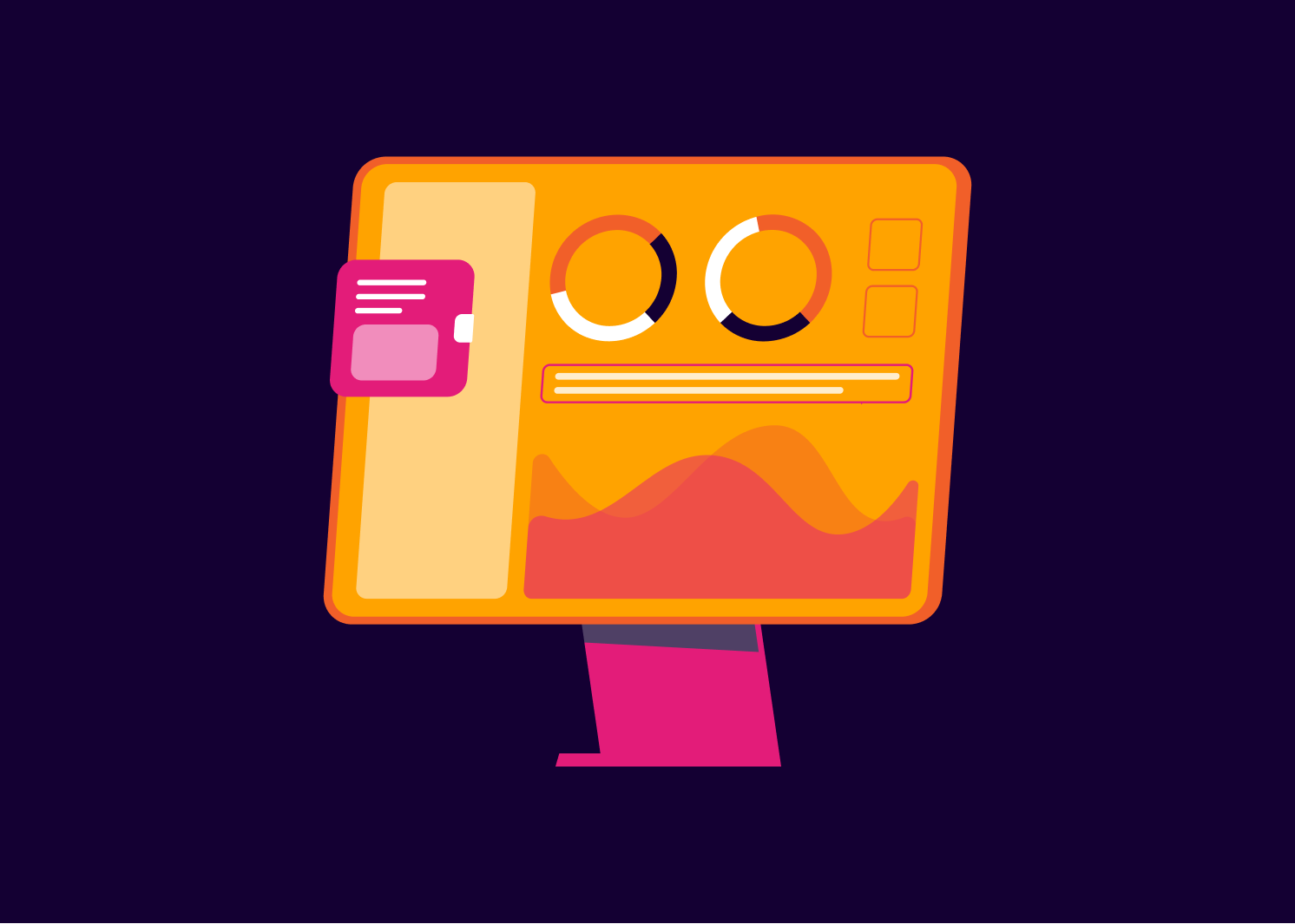
On 24th & 25th February 2021, Workscope attended and sponsored the annual 17th Edition GFMI Model Risk Management conference. This annual conference is one of the premier forums to hear from senior financial industry leaders, regulators, and solution providers on all the latest themes and challenges related to model risk management.
Here are our six key takeaways from this year's conference:
The COVID pandemic has created a sudden impact on models
It is no surprise that shocks to the economic system have created massive swings in economic data such as GDP, unemployment, and commodity prices. While financial models are accepted as an imperfect representation of reality, the volatility in "real world" input data pushes the boundaries for sensitivity and reliability of models. Many organisations are finding that their models require 'adjustments'. This has led to an overall increase in model overlays (overrides) which are used to compensate for the current and unexpected risks and limitation of these models. Firms should have clear and detailed policies for overlays and should distinguish between one-time overlays versus ongoing overlays. The pandemic's impact on models could be stretching overlay policies, and firms should be questioning all overlays. There is a risk that in times of stress, such as we have seen with the pandemic, internal audit groups and model validators may be accepting more ongoing overlays, and the boundary of responsibility between first and second-line responsibilities can become strained.
Artificial Intelligence & Machine Learning models present new challenges and opportunities
The use of artificial intelligence (AI) and machine learning (ML) models is accelerating across the financial services industry. Benefits such as reducing costs, identifying complex relationships, improving model accuracy and performance, are all highly beneficial to financial services firms' performance and competitiveness.
From a senior management perspective, the use of AI and ML presents new challenges for maintaining governance checks and controls. For example, how can organisations ensure that the datasets being used to train models do not introduce bias, which could negatively affect a client outcome? Furthermore, the complexity of machine learning models can introduce a lack of explainability and transparency; this presents challenges for the second and third line of defence responsible for ensuring that models are built and used according to internal policies and procedures. This can be even more challenging where models are provided by third-party vendor's which can operate as black boxes. As the adoption of AI and ML increases, organisations need to plan carefully to ensure that they have the right level of expertise to develop methods and maintain appropriate safeguards for the development, validation, governance, and independent review of models. Ideally, models should alert stakeholders when they are operating outside of their expected ranges and tolerances.
First-line of defence must take ownership and accountability for Model Risk
Many experts agree that the most critical control and risk management responsibilities for model risk reside with the first line of defence. The first line of defence has dual responsibility for revenue-generating activities for the business while also maintaining ownership of the associated risks and controls for the models they use in these activities. If the first line of defence is not heavily involved in the model risk management process, things can get missed, which can introduce risks and undermine the model risk management process for the second and third lines of defence. For example, second-line stakeholders may not have the full knowledge and expertise to effectively challenge the validation of complex models. With the increased use of AI & ML, the challenge of validating models and model explainability is becoming more complex. By proactively engaging and highlighting first-line accountability, the overall risk management process can be strengthened. This ensures that boundaries of responsibility are clear and the second line of defence can perform independent checks and ensure that the appropriate policies and procedures are embedded across the organisation.
How can firms improve first-line risk management?
Improving the first-line risk management function does require management to set the tone and risk-culture at a senior level to ensure that responsibilities and incentives are aligned with the corporate governance goals. Furthermore, leveraging technology for collecting, automating, and reporting on model-risk management tasks can help reduce the manual burden and increase the overall increase effectiveness of the risk management process.
Migrating models away from LIBOR requires careful planning and execution
Many financial institutions are still in the process of removing model references to LIBOR. Institutions have been encouraged to stop referencing the rate by the end of 2021, even though the publication of LIBOR will continue until 30 June 2023. With the pandemic disrupting organisations' priorities and capacity, there is likely going to be increased pressure for completing this migration initiative.
Pre-pandemic, this initiative already presented a variety of operational, compliance, market and compliance risks for institutions. For example, firms need to identify which models are still referencing LIBOR, which can be challenging if there is no complete inventory of models. Models need to be updated with new reference rates, tested and revalidated to ensure they are performing as expected.
Because there is so much uncertainty and because firms are operating under increased pressure, regulators encourage firms to establish contingency plans if the transition does not progress as smoothly as planned.
Automation can augment the MRM process
Automation presents an opportunity in multiple areas such as discovery, validation and documentation. By using high-quality automation tools, MRM teams can expedite the validation process. However, this should be done with caution, and there must be robust controls in place to ensure that firms are not left with a black box or leave the organisation exposed to issues such as key person risk. Therefore, documentation must be a priority as this will safeguard against risks and allow for faster remediation should something go wrong. What a bot or automation script is validating should be clearly documented, including the tolerances, ranges of data etc. The scope and depth of automation processes must be well documented and continuously reviewed.
Collaboration and developing a dialogue with industry peers is becoming increasingly important so that organisations can benchmark the best approaches to automation, especially when looking at newer technologies involving AI and ML.
As the industry looks forward and embraces newer technologies, organisations should still take precautions as there remains a need for the human element and critical judgement, which should not necessarily be eliminated entirely.
Only 9% of companies have an MRM inventory solution that meets their needs
During one of the presentation sessions, experts were asked, "Would you say your firm's current inventory solution meets your firms MRM needs? Only 9% of the experts polled responded Yes. Around half of the respondents were somewhat satisfied with the solution, although they highlighted it could be better. 23% of respondents indicated that they were not satisfied and were actively looking for a better solution.
If this poll is indicative of the financial services landscape, then this sample data suggests there is a considerable gap between what firms need from a model inventory solution and what they are currently have received from internally built or incumbent third-party solution providers.
How can Workscope augment the Model Risk Management process?
The area of model risk management that is often overlooked is the ecosystem in which the models exist. Having good policies and robust processes is half the challenge. Firms need to know where these models have been deployed, who is using them and when they are being used. This can help an organisation understand the operational risk of these models. Model discovery is also crucial to the governance process. You can only govern what you know exists.
For all spreadsheet-based models, Workscope provides a complete automated model inventory (whether it is 50 models or 5 million models), including automatic discovery and real-time reporting of risks and issues. These tools are available to all three lines of defence.
Model inventory
- Maintain a real-time view across the inventory of spreadsheets that exist across the organisation. Achieve this without moving files or disrupting end-users workflow.
Model taxonomy
- Assign owners and classifications to spreadsheet models and quickly establish a standard taxonomy across your model inventory.
Data Lineage. - Get visibility into what data is feeding into models and from which sources.
Dependency Analysis
- Understand which other processes are dependent on the output of models and clearly see the impact of decommissioning models on other files.
- Maintain visibility on data-lineage across the spreadsheet model ecosystem.
Model Integrity
- Take a proactive approach by automatically detecting structural issues or errors within spreadsheet models so corrective action can be taken before its too late.
Stay up to date and read the latest news from Workscope
Related Posts
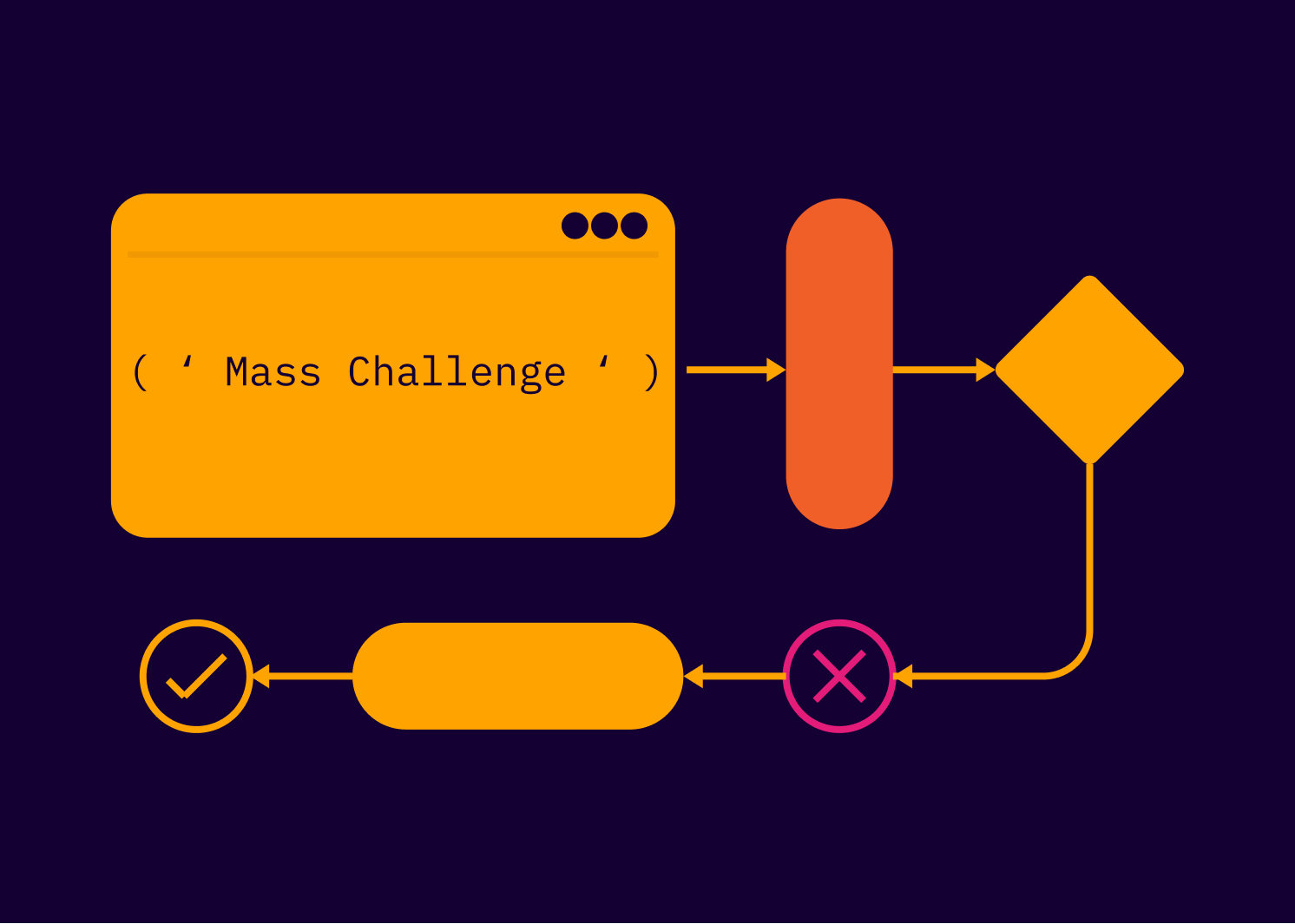
Workscope Joins MassChallenge Fintech 2021 Vertical
Workscope has joined the MassChallenge FinTech 2021 accelerator programme. It is one of only thirty companies accepted from over 380 applicants.

Process Mining vs Task Mining: Understanding the differences & where Workscope fits in
This post explores the differences between process mining and task mining and how Workscope's spreadsheet mining solution can enhance the process optimisation journey.
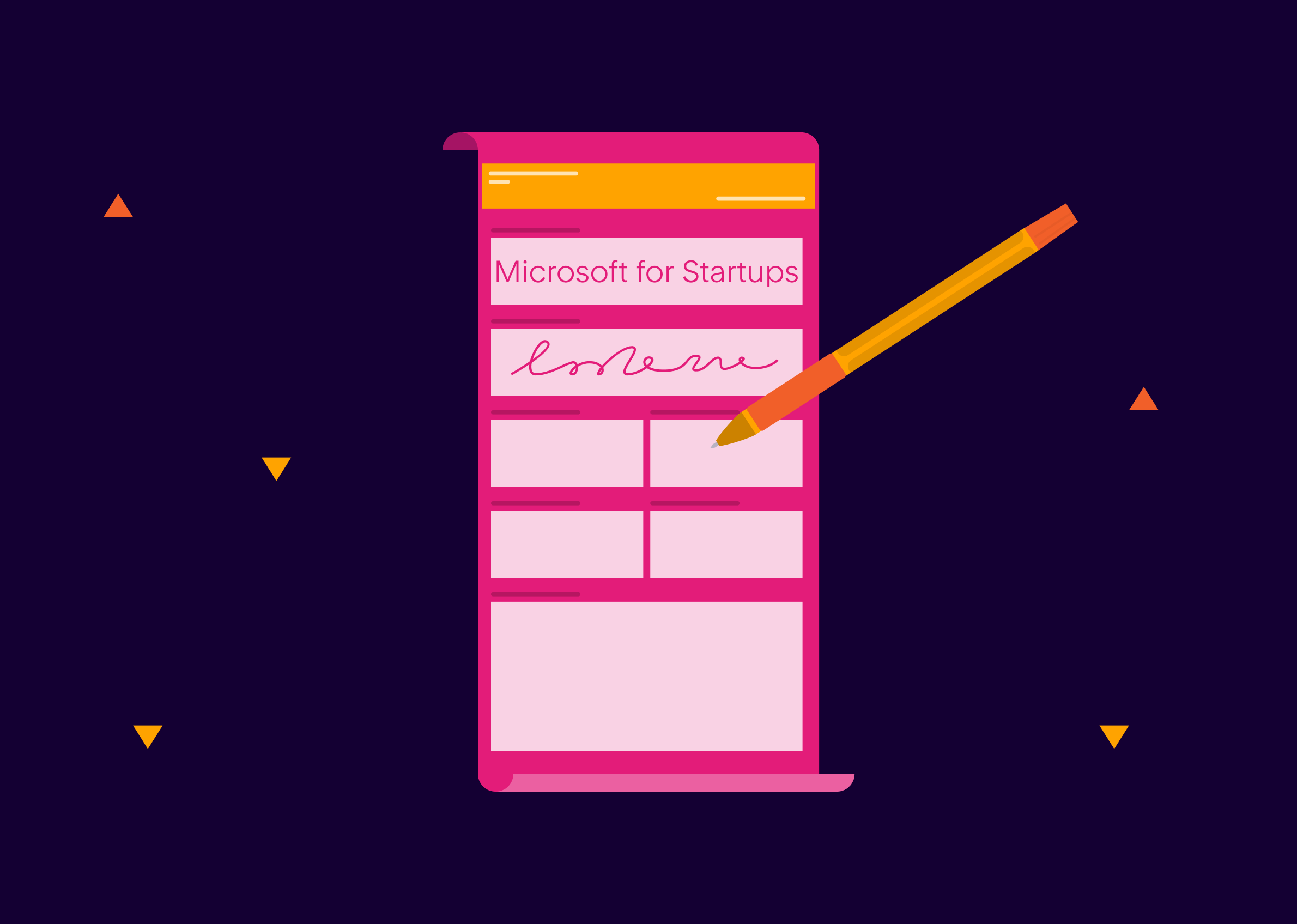
Workscope joins Microsoft for Startups program
Workscope Joins Microsoft for Startups to Scale with Access to Technology and Business Benefits. Discover the benefits of this global startup program.